Artificial Intelligence: A Blessing or a Threat for Humanity?
In
Login if you are already registered
(votes: 1, rating: 5) |
(1 vote) |
PhD in Technical Sciences, Founder, Aigents, Architect, SingularityNET, RIAC Expert
In August 2018, Czech Technical University in Prague simultaneously hosted several conferences on AI-related topics: human-level AI, artificial general intelligence, biologically inspired cognitive architectures, and neural-symbolic integration technology. Reports were presented by prominent experts representing global leaders in artificial intelligence: Microsoft, Facebook, DARPA, MIT and Good AI. The reports described the current status of AI developments, identified the problems facing society that have yet to be resolved, and highlighted the threats arising from the further development of this technology. In this review, we will attempt to briefly identify the main problems and threats, as well as the possible ways to counter these threats.

AI Nationalism and AI Nationalization Are ahead
To begin with, let us provide definitions for some of the terms that are commonly used in conjunction with AI in various contexts: weak, or specialized, AI; autonomous AI; adaptive AI; artificial general intelligence (AGI); strong AI; human-level AI; and super-human AI.
Weak, or specialized, AI is represented by all existing solutions without exception and implies the automated solution of one specific task, be it a game of Go or face recognition with CCTV footage. Such systems are incapable of independent learning for the purpose of solving other problems: they can only be reprogrammed by humans to do so.
Autonomous AI implies a system’s ability to function for protracted periods of time without the intervention of a human operator. This could be a solar-powered UAV performing a multi-day flight from Champs-Elysees in Paris to Moscow’s Red Square or back, independently selecting its route and recharging stops while avoiding all sorts of obstacles.
Adaptive AI implies the system’s ability to adapt to new situations and obtain knowledge that it did not possess at the time of its creation. For example, a system originally tasked with conducting conversations in Russian could independently learn new languages and apply this knowledge in conversation if it found itself in a new language environment or if it deliberately studied educational materials on these new languages.
Artificial general intelligence implies adaptability of such a high level that the corresponding system could, given the appropriate training, be used in a wide variety of activities. New knowledge could either be self-taught or learned with the help of an instructor. It is in this same sense that the notion of strong AI is often used in opposition to weak or specialized AI.
Human-level AI implies a level of adaptability comparable to that of a human being, meaning that the system is capable of mastering the same skills as a human and within comparable periods of time.
Super-human AI implies even greater adaptability and learning speeds, allowing the system to masker the knowledge and skills that humans would never be able to.
Fundamental Problems Associated with Creating a Strong AI
Despite the multitude of advances in neuroscience, we still do not know exactly how natural intelligence works. For this same reason, we do not know for sure how to create artificial intelligence (AI). There are a number of known problems that need to be resolved, as well as differing opinions as to how these problems should be prioritized. For example, Ben Goertzel, who heads the OpenCog and SingularityNET, open-source international projects to create artificial intelligence, believes that all the requisite technology for creating an artificial general intelligence has already been developed, and that the only thing necessary is to combine them in a way that would ensure the necessary synergy [1]. Other experts are more sceptical, pointing out that many of the problems that we will discuss below need to be resolved first. Also, expert estimates for when a strong AI may be created vary greatly, from ten or so years to several decades from now.
On the other hand, the emergence of a strong AI is logical in the framework of the general process of evolution as the emergence of molecules from atoms and cells from molecules, the creation of the central nervous system from specialized cells, the emergence of social structure, the development of speech and writing systems and, ultimately, the nascence of information technology. Valentin Turchin demonstrates the logic behind the increasing complexity of information structures and organizational mechanisms in the process of evolution [2]. Unless humanity perishes first, this evolution will be inevitable and will, in the long run, rescue humankind, as only non-biological lifeforms will be able to survive the inevitable end of the Solar System and preserve our civilization’s information code in the Universe.
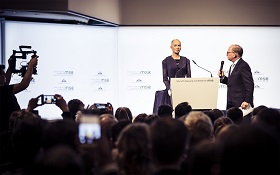
Artificial Intelligence: Time of the Weak
It is important to realize that the creation of a strong AI does not necessarily require the understanding of how the natural intelligence works, just as the development of a rocket does not necessarily require understanding how a bird flies. Such an AI will certainly be created, sooner or later, in one way or another, and perhaps even in several different ways.
Most experts identify the following fundamental problems that need to be solved before a general or strong AI can be created [3]:
- Few-shot learning: systems need to be developed that can learn with the use of a small amount of materials, in contrast to the current deep-learning systems, which require massive amounts of specifically prepared learning materials.
- Strong generalization: creating problem recognition technologies allowing for recognizing objects in situations that differ from those in which they were encountered in the learning materials.
- Generative learning models: developing learning technologies in which the objects to be memorized are not the features of the object to be recognised, but rather the principles of its formation. This would help in addressing the more profound characteristics of objects, providing for faster learning and stronger generalization.
- Structured prediction and learning: developing learning technologies based on the representation of learning objects as multi-layered hierarchical structures, with lower-level elements defining higher level ones. This could prove an alternative solution to the problems of fast learning and strong generalization.
- Solving the problem of catastrophic forgetting, which is pertinent to the majority of existing systems: a system originally trained with the use of one class of object and then additionally trained to recognize a new class of objects loses the ability to recognize objects of the original class.
- Achieving an incremental learning ability, which implies a system’s ability to gradually accumulate knowledge and perfect its skills without losing the previously obtained knowledge, but rather obtaining new knowledge, with regard to systems intended for interaction in natural languages. Ideally, such a system should pass the so-called Baby Turing Test by demonstrating its ability to gradually master a language from the baby level to the adult level.
- Solving the consciousness problem, i.e. coming up with a proven working model for conscious behaviour that ensures effective prediction and deliberate behaviour through the formation of an “internal worldview,” which could be used for seeking optimum behavioural strategies to achieve goals without actually interacting with the real world. This would significantly improve security and the testing of hypotheses while increasing the speed and energy efficiency of such checks, thus enabling a live or artificial system to learn independently within the “virtual reality” of its own consciousness. There are two applied sides to solving the consciousness problem. On the one hand, creating conscious AI systems would increase their efficiency dramatically. On the other hand, such systems would come with both additional risks and ethical problems, seeing as they could, at some point, be equated to the level of self-awareness of human beings, with the ensuing legal consequences.
Potential AI-Related Threats
Even the emergence of autonomous or adaptive AI systems, let alone general or strong AI, is associated with several threats of varying degrees of severity that are relevant today.
The first threat to humans may not necessarily be presented by a strong, general, human-level or super-human AI, as it would be enough to have an autonomous system capable of processing massive amounts of data at high speeds. Such a system could be used as the basis for so-called lethal autonomous weapons systems (LAWS), the simplest example being drone assassins (3D-printed in large batches or in small numbers).

Three Groups of Threats from Lethal Autonomous Weapons Systems
Second, a threat could be posed by a state (a potential adversary) gaining access to weapons system based on more adaptive, autonomous and general AI with improved reaction times and better predictive ability.
Third, a threat for the entire world would be a situation based on the previous threat, in which several states would enter a new round of the arms race, perfecting the intelligence levels of autonomous weapon systems, as Stanislaw Lem predicted several decades ago [4].
Fourth, a threat to any party would be presented by any intellectual system (not necessarily a combat system, but one that could have industrial or domestic applications too) with enough autonomy and adaptivity to be capable not only of deliberate activity, but also of autonomous conscious target-setting, which could run counter to the individual and collective goals of humans. Such a system would have far more opportunities to achieve these goals due to its higher operating speeds, greater information processing performance and better predictive ability. Unfortunately, humanity has not yet fully researched or even grasped the scale of this particular threat.
Fifth, society is facing a threat in the form of the transition to a new level in the development of production relations in the capitalist (or totalitarian) society, in which a minority comes to control material production and excludes an overwhelming majority of the population from this sector thanks to ever-growing automation. This may result in greater social stratification, the reduced effectiveness of “social elevators” and an increase in the numbers of people made redundant, with adverse social consequences.
Finally, another potential threat to humanity in general is the increasing autonomy of global data processing, information distribution and decision-making systems growing, since information distribution speeds within such systems, and the scale of their interactions, could result in social phenomena that cannot be predicted based on prior experience and the existing models. For example, the social credit system currently being introduced in China is a unique experiment of truly civilizational scale that could have unpredictable consequences.
The problems of controlling artificial intelligence systems are currently associated, among other things, with the closed nature of the existing applications, which are based on “deep neural networks.” Such applications do not make it possible to validate the correctness of decisions prior to implementation, nor do they allow for an analysis of the solution provided by the machine after the fact. This phenomenon is being addressed by the new science, which explores explainable artificial intelligence (XAI). The process is aided by a renewed interest in integrating the associative (neural) and symbolic (logic-based) approaches to the problem.
Ways to Counter the Threats
It appears absolutely necessary to take the following measures in order to prevent catastrophic scenarios associated with the further development and application of AI technologies.
- An international ban on LAWS, as well as the development and introduction of international measures to enforce such a ban.
- Governmental backing for research into the aforementioned problems (into “explainable AI ” in particular), the integration of different approaches, and studying the principles of creating target-setting mechanisms for the purpose of developing effective programming and control tools for intellectual systems. Such programming should be based on values rather than rules, and it is targets that need to be controlled, not actions.
- Democratizing access to AI technologies and methods, including through re-investing profits from the introduction of intellectual systems into the mass teaching of computing and cognitive technologies, as well as creating open-source AI solutions and devising measures to stimulate existing “closed” AI systems to open their source codes. For example, the Aigents project is aimed at creating AI personal agents for mass users that would operate autonomously and be immune to centralized manipulations.
- Intergovernmental regulation of the openness of AI algorithms, operating protocols for data processing and decision-making systems, including the possibility of independent audits by international structures, national agencies and individuals. One initiative in this sense is to create the SingularityNET open-source platform and ecosystem for AI applications.
1. Ben Goertzel, Cassio Pennachin and Nil Geisweller, Engineering General Intelligence, Part 1: A Path to Advanced AGI via Embodied Learning and Cognitive Synergy (Atlantis Thinking Machines), Atlantis Press, 2014th Edition // 2014. URL: https://www.amazon.com/Engineering-General-Intelligence-Part-Cognitive/dp/9462390266
2. V. Turchin, The Phenomenon of Science: A Cybernetic Approach to Human Evolution. 2nd edition. Moscow: ETS. 2000, 368 pp. URL: http://refal.net/turchin/phenomenon/
3. 3. M. Iklé, A. Franz, R. Rzepka, B. Goertzel, B. (Eds.), Artificial General Intelligence, 11th International Conference, AGI 2018, Prague, Czech Republic, August 22-25, 2018. // 2018. URL: https://www.springer.com/us/book/9783319976754
4. Stanislaw Lem, Peace on Earth, Operation Eternity Almanac 1988, M.: Mir, 1988. // AI Safety Research: A Road to Nowhere. 2016. URL: https://medium.com/@petervoss/ai-safety-research-a-road-to-nowhere-f1c7c20e8875
(votes: 1, rating: 5) |
(1 vote) |
The Arms Race in Artificial Intelligence and Autonomous Systems has already Begun
AI Nationalism and AI Nationalization Are ahead“Artificial Intelligence” (AI) as the key factor in economic, geopolitical and military power of the coming decades
Artificial Intelligence: Time of the WeakCurrent Status, International Trends, Myths and Prospects of AI Application
Moscow Hosts a Conference on «International and Social Consequences of Using Artificial Intelligence Technologies»The conference brought together experts in artificial intelligence, experts in international affairs, the military, business representatives, government and public figures, and journalists.
Three Groups of Threats from Lethal Autonomous Weapons SystemsSome experts believe that maintaining strategic stability in the coming decades will require a revision of the foundations of the deterrence theory in the multipolar world